Executive summary
It has been 10 years since the discovery of Skimer, the first malware specifically designed to attack automated teller machines (ATMs). At the time, the learning curve for understanding its functionality was rather steep and analysis required specific knowledge of a manufacturer's ATM API functions and parameters, which were not publicly documented.
Before the discovery of Skimer, anti-malware researchers' considered ATMs secure machines containing proprietary hardware, running non-standard operating systems, and implementing a number of advanced protection techniques designed to prevent attacks using malicious code. Researchers eventually discovered that the most popular ATM manufacturers use a standard Windows operating system and add on some auxiliary devices, such as a safe and card reader.
Over time, actors behind some of the newer ATM malware families such as GreenDispenser and Tyupkin realized that there is a generic Windows extension for Financial Services API (CEN/XFS) that can be used to make malware that runs independent of the underlying hardware platform, as long as the ATM manufacturer supports the framework. This malware can trick the machines into dispensing cash, regardless of whether the attacker has a legitimate bank card.
ATM malware has evolved to include a number of different families and different actors behind them, ranging from criminal groups to actors affiliated with nation states. The significance of ATM malware stems from the fact that it can bring significant financial benefits to attackers and as a consequence cause a significant damage to targeted banks, financial institutions and end users.
Now that this type of malware has been around for more than 10 years, we wanted to round up the specific families we've seen during that time and attempt to find out if the different families share any code.
ATM malware overview
Significance
ATM malware provided criminals with a subtler alternative to physically breaking into the safe built into the ATM. Before the appearance of ATM malware, criminals typically had to employ traditional ways of robbing ATMs, often pulling the physical device out of the ground or blowing it to pieces with dynamite. Obviously, these methods would quickly draw the attention of law enforcement and passersby.
Over the past 10 years, we have seen a steady increase in the number of ATM malware samples discovered. Still, the number of discovered samples is very small compared to almost any other malware category.
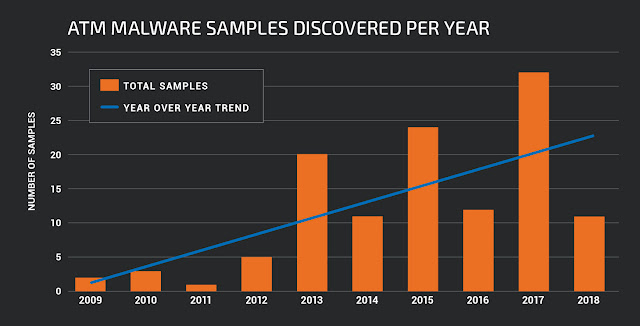
Number of ATM malware samples discovered year over year based on the year of first submission to VirusTotal.
As a digital substitute for dynamite, ATM malware allows criminals to employ money mules and instruct them how to dispense money from targeted ATMs. Typically, it happens by supplying a special authorisation code or card created to authorise the transaction.
Before that, criminals had to infect the targeted ATM to install the code, which more often than not meant that they had to physically open the device to access its optical media reading devices or USB ports.
There have been many reported attacks on various banking organizations throughout the world, but they seem to be more prevalent in Latin America and Eastern Europe, where the ATM infrastructure is older and are not regularly updated with security software or tamper-proof sensors. The damage caused by ATM malware to banks and individuals is rarely disclosed but it likely reaches millions of dollars a year.
ATM malware affects banks and other financial institutions, as well as the reputation of ATM manufacturers and individuals and companies whose account details are stolen in ATM malware attacks.
Classification
There are several different ways we can classify ATM malware families. Based on its functionality, we can classify ATM malware into virtual skimmers and cash dispensers. The purpose of skimmers is to steal card and transaction details and individual PINs if the encryption keys used by pin pad are successfully retrieved.
Cash-dispensing malware uses functions to allow for so-called "jackpotting" of ATMs where money is dispensed by attackers without the authorisation from the bank. But there are malware families that can steal card details and dispense cash.
As far as the installation process is concerned, we again have two major groups. The first one requires the attacker to physically access the device. The second group assumes that the attacker installs malware indirectly, typically by compromising the internal network of the bank and then targeting ATMs using stolen credentials.
These types of malware will also either target specific models of ATMs, or will be more generic. Recently, ATM malware typically deploys generic functions.
The most common framework is the CEN/XFS framework, which allows the developers of the ATM applications to compile and run their code regardless of the ATM model or the manufacturer but there are others, such as Kalignite framework built on top of XFS.
The XFS API contains high-level functions for communicating with the various ATM modules such as the cash dispensing module (CDM), PIN pad (EPP4) or printer. The high-level functions are provided through a generic SDK, while the lower level functions, supplied through service providers, are developed by ATM manufacturers. The architecture is quite similar to Win32 architecture where the developers use the high-level API to communicate with the OS kernel and various device drivers provided by the manufacturers of the individual hardware components.
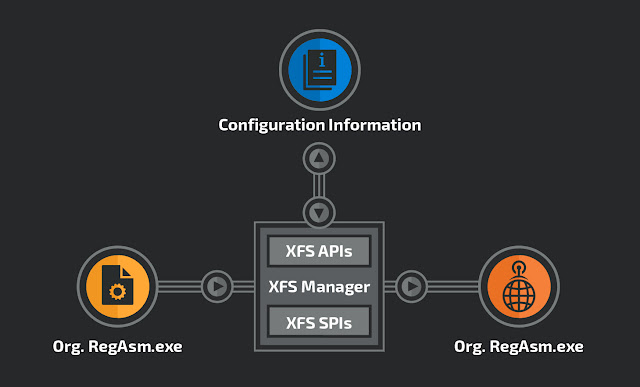
High-level CEN/XFS architecture.
Most ATM samples require physical access to the targeted ATM. ATMs are not typically connected to the internet and communicate with bank's central systems through specialized lines. However, most of the ATMs are connected to internal networks for their maintenance and administration so the second, smaller group of ATM malware may be introduced by compromising the internal network first. This technique requires a higher level of sophistication but potentially brings higher returns if successful.
Some generic hacking tools, such as Cobalt Strike, have reportedly been used for attacking ATMs and the transaction systems. This method has been more commonly used by more advanced groups such as Carbanak, Cobalt Gang and Lazarus (Group 77), whose Fastcash attack affects IBM AIX operating system, which is rarely targeted by malware.
Notable ATM malware families and their functionality
Over the past 10 years, we have seen more than 30 different ATM malware families. In this section, we will briefly describe some of the more notable ones.
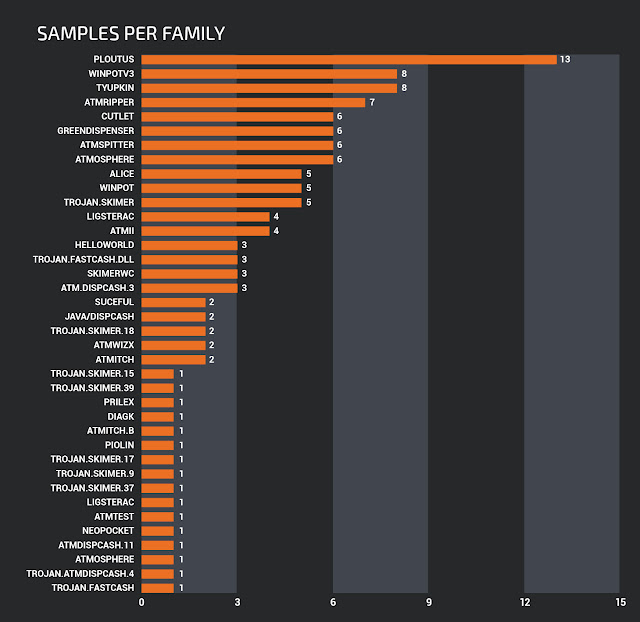
Number of ATM malware samples per family.
Ploutus
Ploutus is the malware family with the largest number of discovered samples. The majority of them having been reported in Mexico. Ploutus is a standard ATM-dispensing malware. The attackers need to be able to access physical ports or a CD-ROM drive to be able to boot from it and modify the ATM system image to install the malware.
Attackers allegedly used newer Ploutus variants to attack some U.S.-based ATMs. Ploutus.D communicates with the ATM using the multi-vendor KAL Kalignite framework, which allows it to work with ATMs from different vendors with minimal changes to its code base.
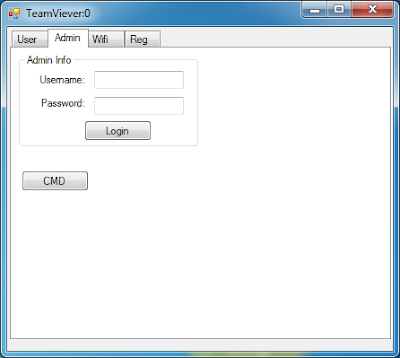
One of the Ploutus variant's interface.
Skimer
Skimer is one of the first ATM attacks, and bears all of the features of well-developed malware. Skimer functions as a virtual skimming device that attempts to steal bank card numbers and details of the account and owner details stored on the magnetic stripe tracks 1 and 2. A recent review of its functionality also indicates that it may also attempt to steal users' PINs by retrieving the encrypted pin pad encryption keys from the system.
Apart from the virtual skimming function, Skimer acts as a backdoor to the ATM functionality for its operators — money mules employed to collect stolen data and dispense cash.
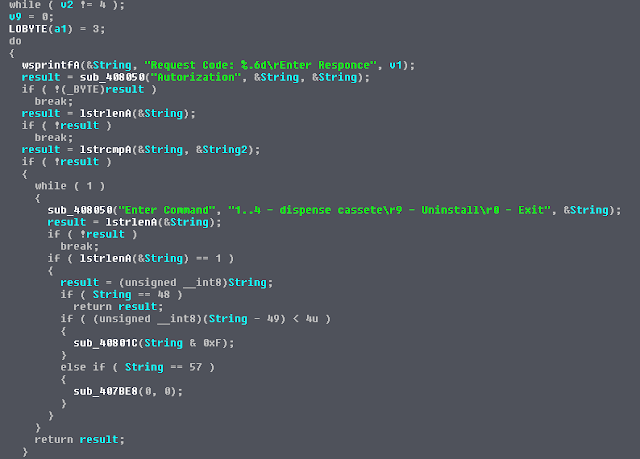
Main code loop for servicing Skimer's operators with cash.
If the user knows the secret code to activate the backdoor, the malware displays a menu, which allows the operator to empty one of the four cash-dispensing modules (CDMs).
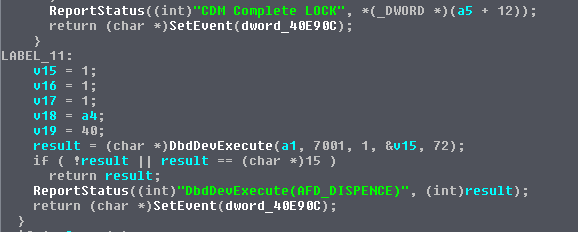
The code locking the dispenser module and dispensing cash.
Most of the other ATM malware families follow a similar principle. The attackers need to be able to physically access the ATM, which requires a key or drilling a hole to access specific ports or devices. Once the malware is deployed, the money mules need a specific code to access the menu and dispense cash.
Tyupkin (Padpin)
The most interesting characteristic of Tyupkin is that it has the ability to limit its operation to specific hours and days of the week. It was reported that some Tyupkin instances can only be used on Sundays and Mondays at night.
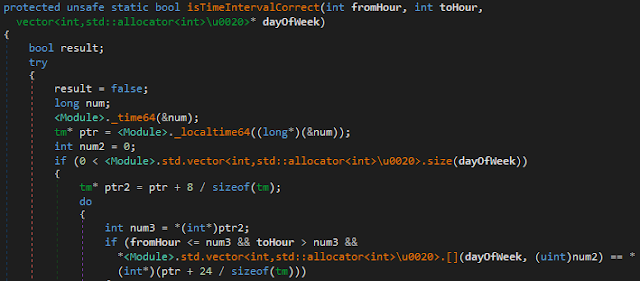
Tyupkin function for checking the hours of operation.
Before dispensing cash, Tyupkin disables any network connections, presumably to prevent administrators from shutting down the ATM if a suspicious activity is detected.
Some members of Tyupkin family are developed using C# and the .NET framework and some using Microsoft Visual C++. The family uses XFS API to manage infected ATMs and dispense cash in multiple currencies. Tyupkin has been active since 2014 and the associated gangs reportedly target Eastern European countries.
Alice
Alice follows a similar pattern to other ATM malware. It is installed by attackers and requires physical access to the system. When the operator launches it, Alice displays a window requiring a PIN.
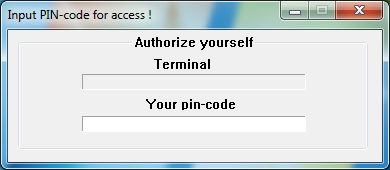
First Alice screen.
If the code is correct, Alice will access the dispenser module and allow the operator to retrieve cash.
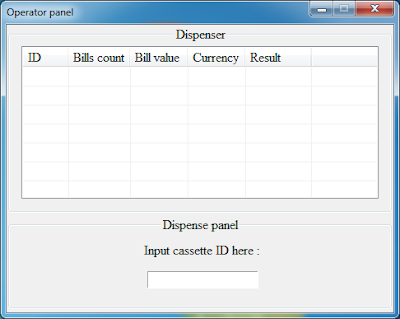
Main Alice UI window.
Cutlet
Cutlet, or Cutlet Maker, has been sold as a do-it-yourself ATM malware kit on some underground markets since 2016. The bundle contains detailed instructions in Russian and English on how to infect systems and how to acquire codes required to dispense cash.
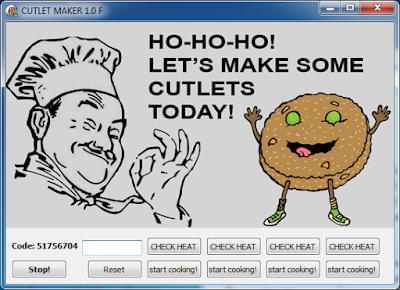
Main Cutlet Maker user interface.
The Cutlet manual details operational security practices required to avoid being caught by law enforcement officers and shows where to drill holes in the ATM enclosure in order to access USB ports of a specific ATM model. The kit also contains a testing application named "Stimulator" for users to practice before they decide to conduct real attacks.
Cutlet follows a similar pattern to the previous ATM malware. The owner of the kit has the ability to generate codes per ATM required for its operation.
Fastcash
The significance of Fastcash malware is its mode of operation and its targeting of IBM AIX operating system. Fastcash consists of a process injector and shared objects presumably injected into the process space of compromised bank payment authorization systems. The malware monitors ISO8583-based transactions using code from a fairly old open-source library for parsing ISO8583 packets.
If an ATM transaction contains the attackers' codes, the data will not be forwarded to the original payment authorisation application and the transaction approval will be sent back to the target ATM system allowing attackers to dispense cash.
This mode of operation is similar to some rootkits, where malware attempts to hide its presence on the system by modifying the responses sent back from the operating system to the application that attempts to list system objects such as files or processes. The returned list is usually modified to remove names of processes that belong to the malware.
Fastcash has been attributed to the Lazarus Group and it is an example of a nation-state-related actor targeting financial systems for the attacker's financial benefit. Fastcash shows a level of sophistication and knowledge that is not seen in other, run-of-the-mill, ATM malware.
Code sharing between families
Thanks to Xylitol and the ATM Cybercrime tracker, it was easy to retrieve a fairly complete ATM malware data set, with the addition of the few files connected with the Fastcash campaign.
The data set contains 121 files and it is well suited for analysis and clustering. Out of 121 files, there are 114 PE files and those were used for clustering using the static analysis techniques. Out of 114 PE files there were 37 packed files which may not be suitable for static analysis techniques and 20 DLLs.
While investigating various methods for clustering, we stumbled upon an interesting book, "Malware Data Science" by Joshua Saxe and Hillary Sanders. This book shows basic and more advanced methods for classifying and clustering malicious files and used some of the ideas to cluster our own set.
In our case, the clustering was conducted by extracting the following attributes of each sample:
- Strings extracted from the file
- Disassembled code from the entry point of the file
- File entropy and the presence of a known packer
- Imported or exported functions
- Embedded resources
After collecting the attributes from each sample, Jaccard distance is calculated for every pair of the files in the set. The Jaccard index is a measure of similarity between two sets. The more similar the two samples are, the higher their Jaccard index will be. The index is a number between 0 and 1. For example, the Jaccard index of 0.5 indicates 50 percent overlap between the two sets.
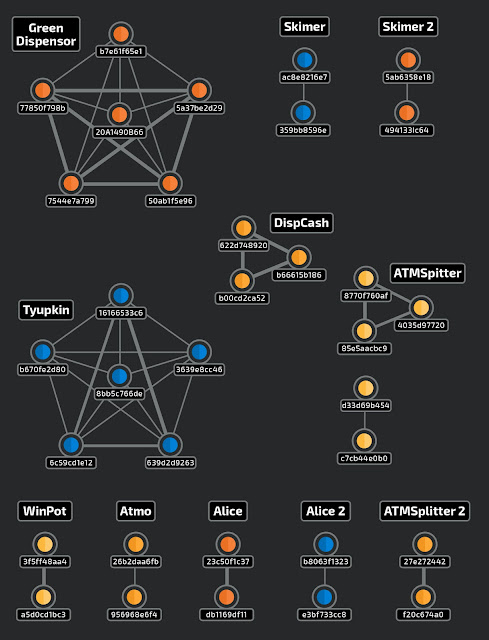
Clusters with Jaccard index threshold of 0.7.
We need to set the threshold required for two samples to be connected as a part of a single cluster. The higher the Jaccard threshold we choose, the more related will be the members of the defined cluster. By varying the threshold we come to the optimal value for our purpose. For example, for correct classification of samples we should choose the value higher than 0.7, and for code sharing purposes, higher than 0.3.
As expected, the results show that as we lower the thresholds we see more clusters appear and some of the clusters show overlap between distinct ATM malware families.
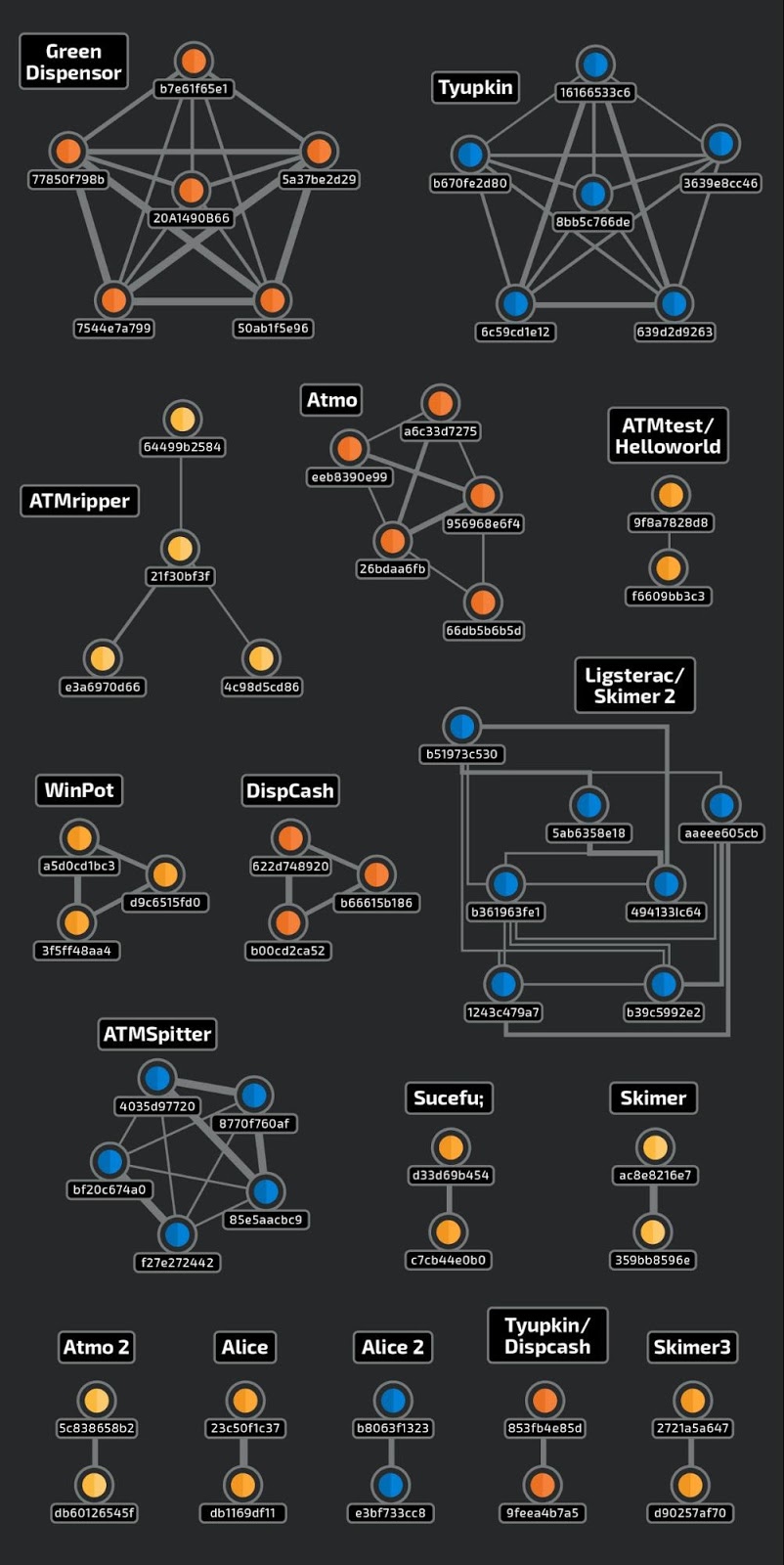
Clusters with Jaccard index threshold of 0.3.
The width of the lines in the graph show how strongly the files in the clusters are related. For example, we see that the members of individual GreenDispenser, Tyupkin or DispCash clusters are very closely related, while mixed Ligsterac/Skimer, Tyupkin/Dispcash and ATMtest/Helloworld clusters show weaker connections that likely indicate some overlap in the malware code.
Protection and detection best practices
When considering protection and detection of attacks with ATM malware, it is important to consider the physical security of ATMs, the security of software running on the system and the security of any segment of the organization's network that communicates with ATMs.
Here are 15 best practices that organizations should follow when considering protection of ATMs networks and successful and timely detection of attacks when they happen.
- Ensure ATMs and all related systems run up-to-date software and the latest operating system versions with the latest security patches applied.
- Disable Windows AutoPlay and configure BIOS to disable the ability to boot software from USB sticks and CD/DVD drives. Set strong BIOS password protection to prevent boot settings from being changed.
- Disable access to the Windows desktop at the ATM, ensure RDP sessions are secured with multiple authentication factors such as Duo Authentication for Windows Logon and RDP.
- Remove any unused services and applications from the system to reduce the attack surface. Implement other measures to harden the underlying ATM operating system.
- Monitor the operation of ATMs, as well as their physical integrity. Look for unusual patterns of resets, communication failures and transaction volume.
- Implement strong encryption between the ATM and the host.
- Ensure access to the ATM cabinet is restricted to authorized persons and that such access is electronically logged.
- Perform a security assessment of ATMs, including their physical locations and any networks connecting to them.
- Ensure that firewalls and anti-malware protection are correctly configured.
- Configure whitelisting solutions or operating system features to allow only known, trusted software to run. Make sure that whitelisting cannot be disabled without generating a remote log entry.
- Prevent unauthorized USB devices from being installed using a device control function.
- Educate employees about how they can avoid introducing malware into operational systems.
- Maintain a physically and logically segmented network environment throughout the organization using segmentation technology such as Cisco TrustSec.
- Ensure visibility over network traffic to ATM systems and payment authorisation servers using technology that enhance network visibility, such as Cisco Stealthwatch.
- Monitor threat intelligence feeds to learn about newly detected ATM malware threats.
Conclusion
ATM malware is a niche area attacks, but it potentially brings significant benefits to actors that successfully manage to deploy it. Over 10 years since the discovery of the first specialized malicious code targeting the Diebold Agilis line of ATMs, we have seen over 30 other malware families with varying degrees of sophistication, complexity and success. Most of the successful attacks are reported in countries where the ATMs are older, such as some Latin American countries and Eastern Europe.
While the majority of actors behind ATM malware seem to be less sophisticated criminal actors, the potential of being able to dispense large amounts of cash also attracts more sophisticated criminal groups such as Carbanak and Cobalt Gang, as well as some state-sponsored actors such as Lazarus.
Although the number of known malware samples for ATMs has been very low there has been a steady increase in the trendline for number of discovered samples year over year.
Financial organizations and banks have to be particularly vigilant when considering protection against malware for ATMs and payment systems. Enterprises and individuals may also experience financial loss due to potential of their card details being used for illegal transactions after being skimmed by ATM malware. Best practices should be followed to ensure the highest possible level of protection and organizations should invest into increasing user awareness about the dangers of ATM malware.
Coverage
Additional ways our customers can detect and block these threats are listed below.
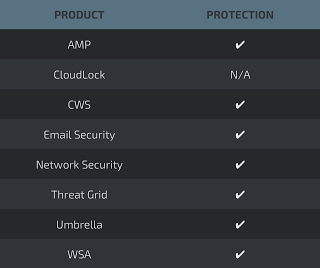
Advanced Malware Protection (AMP) is ideally suited to prevent the execution of the malware used by these threat actors. Below is a screenshot showing how AMP can protect customers from this threat. Try AMP for free here.
Cisco Cloud Web Security (CWS) orWeb Security Appliance (WSA) web scanning prevents access to malicious websites and detects malware used in these attacks.
Email Security can block malicious emails sent by threat actors as part of their campaign.
Network Security appliances such asNext-Generation Firewall (NGFW), Next-Generation Intrusion Prevention System (NGIPS), and Meraki MX can detect malicious activity associated with this threat.
AMP Threat Grid helps identify malicious binaries and build protection into all Cisco Security products.
Umbrella, our secure internet gateway (SIG), blocks users from connecting to malicious domains, IPs, and URLs, whether users are on or off the corporate network.
Open Source SNORTⓇ Subscriber Rule Set customers can stay up to date by downloading the latest rule pack available for purchase on Snort.org.
IOCs
Sha256
Alice
04f25013eb088d5e8a6e55bdb005c464123e6605897bd80ac245ce7ca12a7a70
23c50f1c37b7c55554c282ba1781e9d6279cbbd7bfc5f64772d2e7a8962ebe70
b8063f1323a4ae8846163cc6e84a3b8a80463b25b9ff35d70a1c497509d48539
db1169df116fda46319c4b87607df7b6a5e80b48de5411d47684974ca22dd35a
e3bf733cc85da7421522a0b1ff788d43bcacd02815a88d19426e80de564174b3
ATMii
0ef71569308d44e89bde48096c67caf73ec177c1c970a2fd843fd3a094502d78
5f5d483c1fcd1638b32d11183c5ed5fd36362fb12d62e1d9940b47906733d672
7fac4b739c412b074ee13e181c0900a350b4df9499515febb75008e6955b9674
d74cbd2e39dc0a00dc4c0fb0823c5a86455cdad2be48d32866165c9e5557c3e0
DIAGK
03bb8decefc540bff5b08425adddb404b345452c8adedee0c8af13572891865b
Cutlet
05fae4bef32daf78a8fa42f8c25fdf481f13dfbbbd3048e5b89190822bc470cd
4a340a0a95f2af5ab7f3bfe6f304154e617d0c47ce31ee8426c70b86e195320c
c18b23cc493f89d73a2710ebb177d54beafe0edf0e17cc79e28d9efdfb69a630
d1a0b2a251fa69818784e8937403c18f09b2c37eead80ba61a3edf4ac2b6b7ff
d4a463c135d17239047ad4151ab2f2d084e223970e900904ecedabc0fd916545
fe1634318e27e3af856506d49a54d1d12e1cf650cbc31eeb0c805949edc8fc85
Piolin
5f4215368817570e7a390c9f6e265a7db343c9664d22008d5971dac707751524
Prilex
d10a0e0621a164fad0d7f3690b5d63ecb9561e5ad30a66f353a98395b774384e
WinPot
0720db2469a61d41c1e67a8f32020927a32422a5d58067bb328a2ff407e14e98
3f5ff48aa4dc2c1af3deeb33a9cc576616dad37156ae9182831b1b2a5ae4ae20
a5d0cd1bc33f44d25695ebd6530757180f4fc4d87a1658ee2f0d8fc42d09fb80
c3a5c8e9195163cef8e0e70bd8f3d49c8048e37af7c969341e1753aee63df0ae
d9c6515fd0fb3cd14b4bb4d11ecda78602d17f370780a4b9ee006a9830106213
ATMitch
1065502d7171df7be3776b839410a227c540cd977e5e856bbbcd837b0872bdb6
ea5ebd1e5f98e10b1e7c834dd54707ad06772bccb4179cae7e50c7e6e772a1ab
ATMtest
9f8a7828d833ed7f28f9f5ceaf1c073c6de0645172b8316d86edc16c84b61c4f
ATMWizX
7bd2c97ac5027c360011dc5aa8f2371cd934f73e885e41f7e80152332b3af1db
a4b42f503090cd3cd53963ddaf0be3e4eeedbd81ff02664668e68612816e727f
Ploutus
0106757fac9d10a8e2a22dce5337f404bfa1c44d3cc0c53af3c7539888bc4025
04db39463012add2eece6dfe6f311ad46b76dae55460eea30dec02d3d3f1c00a
0971c166826163093093fb199d883f2544055bdcfc671e7789bd5088992debe5
0e37b8a6711a3118daa1ce2e2f22c09b3f3c6179155b98215a1d96a81c767889
34acc4c0b61b5ce0b37c3589f97d1f23e6d84011a241e6f85683ee517ce786f1
398e335f2d6379771d86d508a43c567b4156104f89161812005a6122e9c899be
62b61f1d3f876300e8768b57d35c260cfc60b768a3e430725bd8d2f919619db2
7fd109532f1e49cf074be541df38e0ce190497847fdb5588767ca35b9620a6c2
aee97881d3e45ba0cae91f471db78aded16bcff1468d9e66edf9d3c0223d238f
c8d57b32ab86a3a97f89ae7f1044a63cca2b58f748bed250a1f9df5c50fc8fbb
d93342bd12ef44d92bf58ed2f0f88443385a0192804a5d0976352484c0d37685
d99339d3dc6891cdd832754c5739640c62cd229c84e04e9e3cad743c6f66b1b9
e75e13d3b7a581014edcc2a397eaffbf91c3e5094d4afd81632d9ad872f935f4
Suceful
c7cb44e0b075cbc90a7c280ef8f1c69e8fe06e7dabce054b61b10c3105eda1c4
d33d69b454efba519bffd3ba63c99ffce058e3105745f8a7ae699f72db1e70eb
Tyupkin
16166533c69f2f04110e8b8e9cc45ed2aeaf7850fa68845c64d92ff907dd44f0
3639e8cc463922b427ea20dce8f237c0c0e82aa51d2502c48662e60fb405f677
639d2d926325275cb023014d0b446d03f1dcc8526bff1aa72373e27d78a6a674
646433de5c56fdbc7e6e934a05e9e99012ef39a0ed6cc4bdb1d984cd4435379e
6c59cd1e12bc1037031af48b934e9398fc85efb2a067d03b6a100dd8423e5d9b
853fb4e85d8b0ad7c156ad6d3fc4b0340c8b29fa0548a3df758e7845ba8b23ae
8bb5c766de0a73dc0eff7c9fce086565b6220465185e258c21c5b9dfb0bef51d
b670fe2d803705f811b5a0c9e69ccfec3a6c3a31cfd42a30d9e8902af7b9ed80
SkimerWC
dff7ee95100ffaec5848a73a7b306eaaee94ae691dfccff9fe6ce0a8f3b82c56
e267fb3044c31256f06dd712c7aeae97ad148fd3157995a7e536e5473c1a2bc0
e78e6155b8dfd206ba5a5e7253409891bfed1b943d217e0fbc416a25fa761580
ATMitch.B
66db5b6b5dc51de7e5380f214f703bdc69ab3c3bec7c3b67179940a06560f126
ATMripper
21f3c0bf3fc05685ec5b7bf3c98103761894d7c6783c2c12afae958eb103598e
22db6a994eb057715b499c5641cc608fb0380aeea25f78180436c35ecd81ce7d
3d8c7fb9e55f96cf3073b321ee5e59ff2189d70b0662bc0b88990971bc8b73d8
4c98d5cd865d7fe2f293862fae42895045e43facfdd2a3495383be4ddbb220dc
64499b2584d239380ffecf07e94167e0414c4bb5438620659fe37d595ef3f361
cc85e8ca86c787a1c031e67242e23f4ef503840739f9cdc7e18a48e4a6773b38
e3a6970d66bc4687b21381353826fabd469007c869efc711fdd0e4711aa77ffc
Ligsterac
1243c478a7145fa08a03200611fcf5fae9bb58039c5069ef93e150d53cf22524
377f85562e9ec16cae8fed87e43b6dd230eaa6e1c8f2732f5096f1ec951f045a
aaeee605cb1850dd81da8990fe4115fe85e5d4eb84ddaf2fa8d0b21afdc2b293
b361963fe11b149afc526a6e0656c08226f943bdba0f2c7c0a7640fba09afce8
e130bc1603893155d87946a430b6d6ad167760cde24aa2834c61dd0eace30e8e
NeoPocket
85652bbd0379d73395102edc299c892f21a4bba3378aa3b0aaea9b1130022bdd
Atmosphere
26b2daa6fbf5ec13599d24e6819202ddb3f770428d732100be15c23be317bd47
5c838658b25d44edab79a4bd2af7c56bef96768b93addbbaaaea36da604fca62
956968e6f4bf611137ea0e747891ba8dc200ca809c252ef249294912fb3dbe3c
a6c33d7275c46397593f53ea136ea8669794f4d787044106594631c07a9ee71d
d60126545fa68b14c36cd4cffa3f81ed487381482582acbba786fa88884f636b
eeb8390e885612e1f0b8f8922baa4ebc9ba420224b30370d08b45f3453949937
ATMSpitter
4035d977202b44666885f9781ac8755c799350a03838ff782eb730c0d7069958
85e5aacbc9113520d93f1d9d73193c3501ebab8032661052d9a66348e204cde6
8770f760af320d30681a4eb4ded331eab2481f54c657aac607df8babe8c11a6b
bf20c674a0533e7c0d825de097629a96cb42ae2d4840b07dd1168993d95163e8
c5b43b02a62d424a4e8a63b23bef8b022c08a889a15a6ad7f5bf1fd4fe73291f
e372631f96face11e803e812d9a77a25d0a81fa41e4ac362dc8aee5c8a021000
f27e27244233f2bb5b02412d4b05315625928adaa340708e91d61ad3bce54bf6
HelloWorld
2de4a510ee303c04c8d7bd59b7987b22c3471c9f4ba69b5f83ba36de88b63a8d
867991ade335186baa19a227e3a044c8321a6cef96c23c98eef21fe6b87edf6a
f6609bb3c3197ace26ebdeb372ba657ac84b05a3e9e265b5211e1ea42da70dbe
Java/Dispcash
0149667c0f8cbfc216ef9d1f3154643cbbf6940e6f24a09c92a82dd7370a5027
ef407db8c79033027858364fd7a04eeb70cf37b7c3a10069a92bae96da88dfaa
Trojan.Skimer
2721a5a6478bfff2c5de0d105623ba5f411401bbd92bd3e2bee4c51c2d12f5a8
4941331c64e0389d5ec966122ef71a99d8f9830f13e9afa758e03275f896c2eb
5ab6358e1886655257c437ebad71b98a6575313b2f9327359661aac5d450c45a
653701d02c5d8d39b3da9b0848d20921cd65ea28e77c8e9254e222601264bcc6
d90257af70401984d5d41dd057114df88566d00329874ced3103a6f8cd1991e5
GreenDispenser
20a1490b666f8c75c47b682cf10a48b7b0278068cb260b14d8d0584ee6c006a5
50db1f5e9692f217f356a592e413e6c9cb31105a94efc70a5ca1c2c73d95d572
5a37be2d298145b766ba54616677d802cfabc62e3b9be2ffb6d4719d3f8143e9
7544e7a798b791cb36caaa1860974f33d30bc4659ceab3063d1ab4fd71c8c7e0
77850f738ba42fd9da299b2282314709ad8dc93623b318b116bfc25c5280c541
b7e61f65e147885ec1fe6a787b62d9ee82d1f34f1c9ba8068d3570adca87c54f
ATM.DispCash.3
622d7489208578eaaaae054a07e16b4b8c91a3fde6e61d082a09aee5a1b1f829
b00cd2ca5247c93e3a40f73006051bbfada3b1bc73c4d44105384824bb60131d
b66615b186bf7067cdb937220f86b1d9411351e0b06ee8d02cf6c5358348e884
9feea4b7a5b438335353bb4eac82f8f2a16232a90b7cddbf77dc73dd451e9a6e
6efedf9bde951ad6c3e240ec498767bb693ecc8fa62040e624c5a7fa21c5bdaa
Trojan.Fastcash
d465637518024262c063f4a82d799a4e40ff3381014972f24ea18bc23c3b27ee
ca9ab48d293cc84092e8db8f0ca99cb155b30c61d32a1da7cd3687de454fe86c
10ac312c8dd02e417dd24d53c99525c29d74dcbc84730351ad7a4e0a4b1a0eba
3a5ba44f140821849de2d82d5a137c3bb5a736130dddb86b296d94e6b421594c
Skimer
34e7060e7a0c0ba24fcb55c641e5b586cef744e10ebd5a9f73ecd2ed2f4e9c1f
b51973c530802ae19df8ac4d9643fc3317952242d9d42f951e094c72d730dd66
359bb8596e4befafdaca706630bec598400694305622c116acdfa59074f1858e
ac8e8216e71e078198ef67d4cb48118767d0696610a02137492814422153d3c6
7888e9a27b27f026f09997414504be5822f35b69ddec826eb2a56f6347e2d147
cde6f7fb2fbdefffe22a012295ab157cffc07cab26ba0e34ced0bae484355187
b39c5992c2cb70c76c82d6fba3cc0b7972c2f9b35227934b766e810f20a5f053
WinPotv3
009b677564b3ebb0831171edf3fb0deb0fa3b0010b74586e01d8df4af965ef3f
1d6508cbe5f7ccaa991572f05aef52bab8a59851ca9a4367605a9637b10ae081
20fb2edfcece271f87d006e263c4a6de48ed518901211a76dc38aac43e1b9d19
6670ccc940cca6983340dbce1a9bbce7b49643ac924e18ca25def8b632b70720
70cc5070ce058682c1d44cef887c0ec8a50dba6b717802c5a8f2c8f2ed377c13
8d7f932d8236671018c5cd02781301134aa6df315253f7a56559350d2616ff8e
b57bc410683aba4c211e407320e6b7746ce25e06d81ddf480711228efd921a6c
e2c87bca353016aced41305ddd66ee7430bf61a20c0f4c8c0f0650f006f05160